Extension for Scikit-learn TSNE example
[1]:
from timeit import default_timer as timer
from sklearn import metrics
from sklearn.datasets import make_blobs
import matplotlib.pyplot as plt
%matplotlib inline
import warnings
warnings.filterwarnings("ignore")
Generate the data
[2]:
x, y = make_blobs(n_samples=20000, centers=4, n_features=100, random_state=0)
Patch original Scikit-learn with Extension for Scikit-learn
Extension for Scikit-learn (previously known as daal4py) contains drop-in replacement functionality for the stock Scikit-learn package. You can take advantage of the performance optimizations of Extension for Scikit-learn by adding just two lines of code before the usual Scikit-learn imports:
[3]:
from sklearnex import patch_sklearn
patch_sklearn()
Extension for Scikit-learn* enabled (https://github.com/uxlfoundation/scikit-learn-intelex)
Extension for Scikit-learn patching affects performance of specific Scikit-learn functionality. Refer to the list of supported algorithms and parameters for details. In cases when unsupported parameters are used, the package fallbacks into original Scikit-learn. If the patching does not cover your scenarios, submit an issue on GitHub.
Training TSNE algorithm with Extension for Scikit-learn for generated dataset
[4]:
from sklearn.manifold import TSNE
params = {"n_components": 2, "random_state": 42}
start = timer()
tsne = TSNE(**params)
embedding_intelex = tsne.fit_transform(x)
time_opt = timer() - start
print(f"Extension for Scikit-learn time: {time_opt:.2f} s")
print(f"Extension for Scikit-learn. Divergence: {tsne.kl_divergence_}")
Extension for Scikit-learn time: 12.63 s
Extension for Scikit-learn. Divergence: 4.289110606110757
### Train the same algorithm with original Scikit-learn In order to cancel optimizations, we use unpatch_sklearn and reimport the class TSNE.
[5]:
from sklearnex import unpatch_sklearn
unpatch_sklearn()
Training algorithm with original Scikit-learn library for generated dataset
[6]:
from sklearn.manifold import TSNE
params = {"n_components": 2, "random_state": 42}
start = timer()
tsne = TSNE(**params)
embedding_original = tsne.fit_transform(x)
time_original = timer() - start
print(f"Original Scikit-learn time: {time_original:.2f} s")
print(f"Original Scikit-learn. Divergence: {tsne.kl_divergence_}")
Original Scikit-learn time: 37.66 s
Original Scikit-learn. Divergence: 4.2955403327941895
Plot embeddings original scikit-learn and Extension
[7]:
colors = [int(m) for m in y]
[8]:
for emb, title in zip(
[embedding_intelex, embedding_original],
["Extension for Scikit-learn", "Original scikit-learn"],
):
plt.scatter(emb[:, 0], emb[:, 1], c=colors)
plt.title(title)
plt.xlabel("x")
plt.ylabel("y")
plt.show()
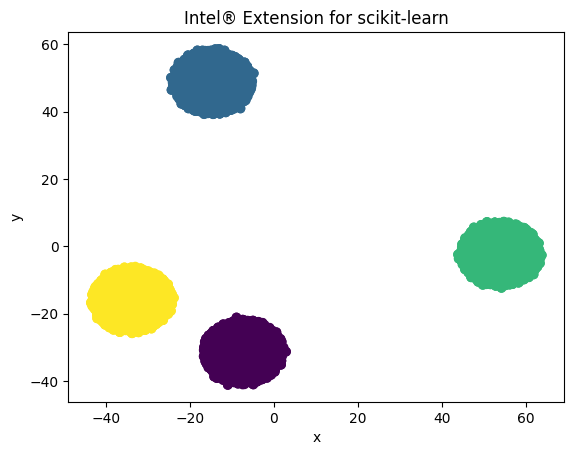
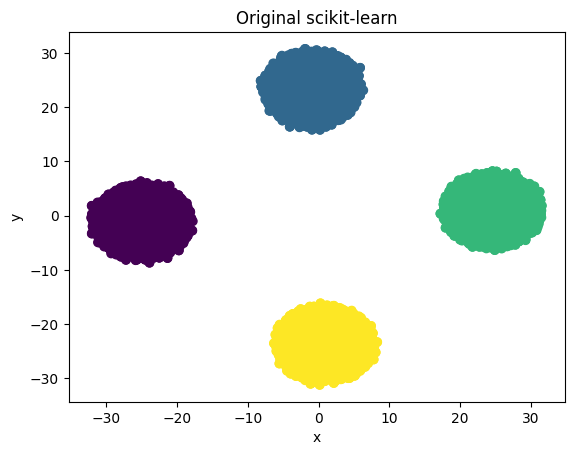
[9]:
f"Speedup for this run: {(time_original/time_opt):.1f}"
[9]:
'Speedup for this run: 3.0'